
“Thought is only a flash in the middle of a long night. But this flash means everything…”
– Henri Poincaré (1854-1912)
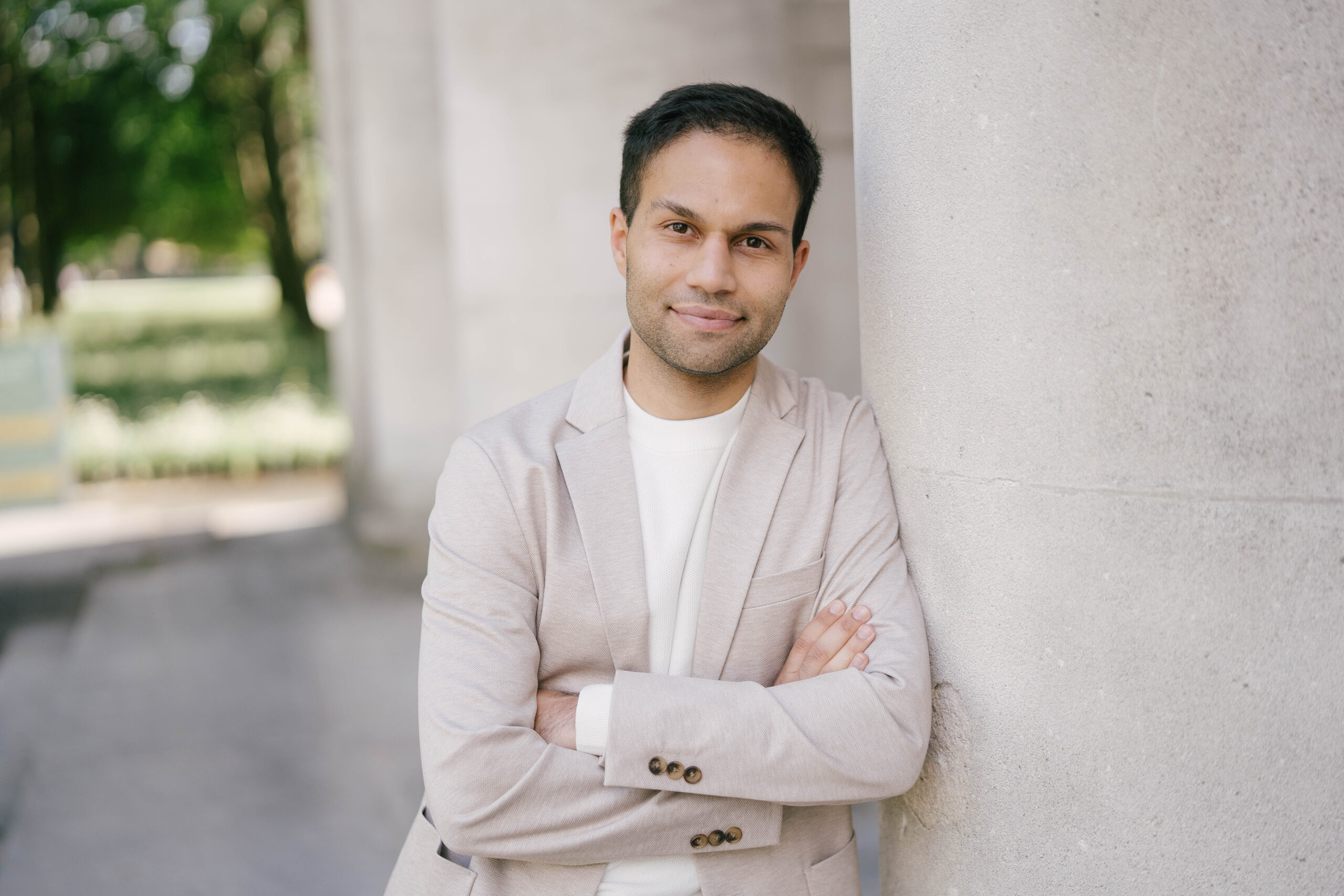
Karim Barigou
From September, I will start my role as Professor of Actuarial Science at the Louvain School of Management (LSM) and the Institute for Multidisciplinary Analysis and Quantitative Modeling (IDAM) at Université catholique de Louvain (UCLouvain).
My research interests are in life insurance and its interplay with quantitative finance. My recent work covers diverse areas such as mortality modelling with pandemics and climate effects, longevity risk management, pricing and hedging variable annuities, and the application of statistical learning techniques in life insurance.
Previously, I was Professor in the School of Actuarial Science at Université Laval (Quebec, Canada). I also worked as a postdoctoral researcher at ISFA, University Lyon 1 (2019-2022), focusing on longevity risk online detection and model selection. I earned my Ph.D. in Business Economics from KULeuven in 2019, where my research under the supervision of Jan Dhaene focused on combining market-consistent and actuarial valuation methods for life insurance liabilities.
Open-Source SofTware
StanMoMo: An R package for Bayesian Mortality Modelling with Stan
Glad to share a new R package for Bayesian Mortality Modelling. The package provides functions to fit and forecast standard mortality models (Lee-Carter, APC, CBD, etc) in a full Bayesian setting. The package also includes functions for model selection and model averaging based on leave-future-out validation.
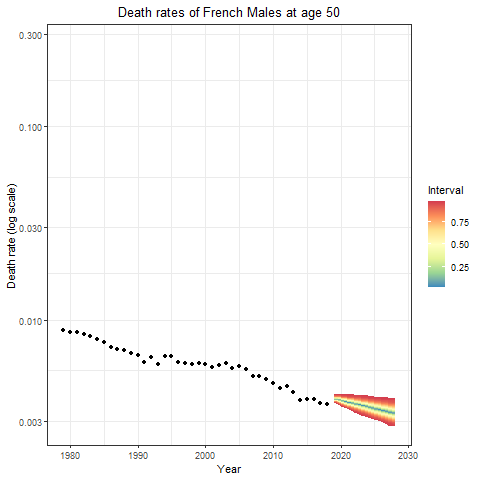
Recent PaperS
Expected Utility Optimization with Convolutional Stochastically Ordered Returns
This paper expands the theoretical framework by considering investment returns modeled by a stochastically ordered family of random variables under the convolution order, including Poisson, Gamma, and exponential distributions. Utilizing fractional calculus, we derive explicit, closed-form expressions for the derivatives of expected utility for various utility functions, significantly broadening the potential for analytical and computational applications. We apply these theoretical advancements to a case study involving the optimal production strategies of competitive firms, demonstrating the practical implications of our findings in economic decision making.
Bayesian mortality modelling with pandemics: a vanishing jump approach
This paper extends the Lee-Carter model to include vanishing jumps on mortality rates, where the impact is highest in the beginning and then gradually diminishes. Existing models either account for transitory jumps over one period or permanent shifts. However, there is no literature on estimating mortality time series with jumps that have a vanishing effect over a small number of periods, as is typically observed in pandemics. Using COVID-19 data, our proposed Bayesian model outperforms transitory shock models in terms of in-sample fit, thus providing a more comprehensive representation of mortality rates during a pandemic.

Actuarial-consistency and two-step actuarial valuations: a new paradigm to insurance valuation
Motivated by solvency regulations, the recent focus has been towards financial risks and the so-called market-consistent valuations. In this situation, the financial market is the main driver and actuarial risks only appear as the ‘second step’. This paper goes against the tide and introduces the concept of 𝒂𝒄𝒕𝒖𝒂𝒓𝒊𝒂𝒍-𝒄𝒐𝒏𝒔𝒊𝒔𝒕𝒆𝒏𝒕 valuations where actuarial risks are at the core of the valuation. We propose a 𝒕𝒘𝒐-𝒔𝒕𝒆𝒑 𝒂𝒄𝒕𝒖𝒂𝒓𝒊𝒂𝒍 𝒗𝒂𝒍𝒖𝒂𝒕𝒊𝒐𝒏 that is first driven by actuarial information and is actuarial-consistent. We also provide a detailed comparison between actuarial-consistent and market-consistent valuations

Bayesian model averaging for mortality forecasting using leave-future-out validation
Relying on one specific model can be too restrictive and lead to some well documented drawbacks including model misspecification, parameter uncertainty and overfitting. In this paper, we consider a Bayesian Negative-Binomial model to account for parameter uncertainty and overdispersion. Moreover, model averaging based on out-of-sample is considered as a response to model misspecifications and overfitting. Overall, we found that our approach outperforms the standard Bayesian model averaging in terms of prediction performance and robustness.

Parsimonious Predictive Mortality Modeling by Regularization and Cross-Validation with and without Covid-Type Effect
Most mortality models are very sensitive to the sample size or perturbations in the data. In this paper, we show how regularization and cross-validation can be used to smooth and forecast the mortality surface. In particular, our approach outperforms the P-spline model in terms of prediction and is much more robust when including Covid-type effect.

Insurance valuation: A two-step generalized regression approach
There is still an open debate on how to appropriately define a "fair" value and a risk margin for long-term insurance liabilities. In this paper, we discuss how solvency constraints can be efficiently included in the hedging process and the risk margin.

Pricing equity-linked insurance by neural networks
In this paper, we price a portfolio of equity-linked contracts in a general incomplete actuarial-financial market. We show that the pricing problem can be efficiently handled by the use of neural networks.
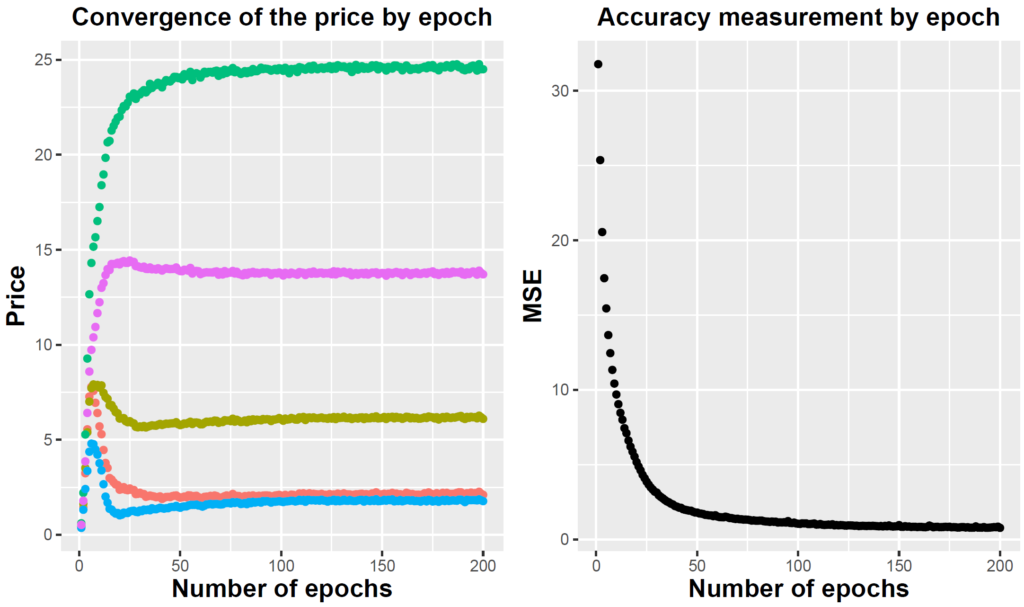